Edge AI vs Cloud AI: Best AI Architecture for Business Use in 2025
Wondering whether Edge AI or Cloud AI is the right infrastructure for your business? Here’s how to decide.
With AI quickly moving from hype to necessity, your choice between Edge AI and Cloud AI isn’t just technical—it’s strategic. The wrong decision today can mean higher costs, slower systems, or even security risks tomorrow.
In this blog, you’ll learn what each AI model offers, how they compare in real-world scenarios, and which one will best support your long-term growth. We’ll break down their key differences, performance metrics, use cases, and even explore a hybrid option that could offer the best of both worlds.
Table of Contents:
- Edge AI vs Cloud AI Architecture at a Glance
- Why Choosing the Right AI Infrastructure (Edge vs Cloud) Matters
- Edge AI Benefits for Business in 2025
- Cloud AI Advantages for Scalable Enterprise AI in 2025
- Edge AI vs Cloud AI: Key Differences for Business
- How to Choose Between Edge and Cloud AI for Business
- Real-World Example: Manufacturing Visual Inspection
- Hybrid AI: The Best of Both Worlds?
- Frequently Asked Questions About Edge AI vs Cloud AI
Edge AI vs Cloud AI Architecture at a Glance
Feature | Benefits of Edge AI | Benefits of Cloud AI |
Data Processing | Local (on-device) | Remote (cloud servers) |
Latency | Ultra-low, real-time | Variable, depends on connection |
Security & Privacy | High – data stays local | Moderate – data transmitted to external servers |
Connectivity | Works offline | Requires internet |
Scalability | Limited by hardware | Virtually unlimited |
Best For | Real-time actions, compliance-heavy industries | Big data, model training, centralized operations |
Bottom Line: Choose Edge AI for instant, local decision-making and privacy control. Go with Cloud AI for scale, analytics, and complex data processing. Many businesses thrive with a hybrid model combining both.
Why Choosing the Right AI Infrastructure (Edge vs Cloud) Matters
AI isn’t optional anymore. It’s becoming the foundation of digital transformation across industries—automating decisions, optimizing operations, and unlocking new business models.
But AI isn’t one-size-fits-all. Your infrastructure choice directly impacts:
- Latency: how fast decisions happen
- Security: how data is handled
- Scalability: how you grow without breaking things
- Cost: both upfront and ongoing
Choosing between Edge and Cloud AI is no longer a conversation just for IT departments. These infrastructure decisions now shape everything from customer experience and data governance to product delivery timelines. For business leaders, aligning your AI strategy with your operational needs from day one helps avoid costly rework or scaling barriers later. Whether your priority is data sovereignty, real-time analytics, or low infrastructure overhead, your choice today will define your speed and resilience tomorrow.
If you’re planning to integrate AI into your operations, this decision is the cornerstone.
Read More: Implementing AI: A Step-by-Step Guide for Startups
Edge AI Benefits for Business in 2025
Edge AI refers to artificial intelligence systems that process data directly on local devices (like cameras, sensors, or gateways), without needing to send everything to the cloud.
Tools like NVIDIA Jetson or Intel Movidius are powering real-time edge deployments in retail, manufacturing, and healthcare.
Advantages of Edge AI
- Edge AI delivers ultra-low latency for real-time decisions without sending data to the cloud.
- Offline capabilities: Works in areas with limited or no connectivity.
- Improved privacy: Data stays local, reducing the risk of breaches.
- Lower bandwidth: Cuts down the amount of data sent to external servers.
Common Edge AI Use Cases
- Manufacturing: Defect detection in real time on the assembly line.
- Retail: Smart shelves that track inventory and shopper behaviour.
- Transportation: Autonomous vehicles processing sensor data locally.
- Healthcare: On-device diagnostics for wearables or imaging tools.
Edge AI isn’t just a technical shift—it’s a competitive one. For businesses that operate in fast-paced or bandwidth-limited environments, the ability to process data locally can unlock major advantages. Imagine industrial machinery adjusting itself in milliseconds or smart retail systems responding instantly to customer movement. These aren’t futuristic concepts—they’re live applications driving efficiency, reducing errors, and safeguarding sensitive data. For companies prioritising control and speed, Edge AI delivers results at the point of action.
Read More: Unlocking AI Potential: A Strategic Approach to AI Adoption for Businesses
Cloud AI Advantages for Scalable Enterprise AI in 2025
Cloud AI uses centralized servers to process data, train models, and run AI tasks. Services like Google Cloud AI, AWS SageMaker, and Microsoft Azure are examples of Cloud AI platforms.
Popular cloud platforms like AWS SageMaker, Google Vertex AI, and Microsoft Azure Machine Learning enable scalable model training and deployment.
Advantages of Cloud AI
- High processing power: Handle massive datasets and complex models.
- Easy scalability: Add more compute without hardware limitations.
- Central management: Everything can be accessed and monitored remotely.
- Integrated tools: Seamless connection with other enterprise cloud tools.
Common Cloud AI Use Cases
- Big data analysis: Customer behaviour trends across millions of transactions.
- Marketing automation: AI-driven campaign optimization.
- Enterprise software: Smart document handling, NLP, and business forecasting.
- Healthcare: Predictive analytics using centralized health records.
Cloud AI remains the default choice for companies looking to centralise their operations, handle vast datasets, and evolve quickly with scalable resources. It’s particularly valuable when working across multiple locations or departments, offering a consistent, remotely managed platform for deploying intelligence. Think of it as the brain in the cloud, constantly learning from massive inputs and broadcasting smarter outputs to your tools and teams. For businesses aiming to build or refine AI models at scale, Cloud AI provides the horsepower and flexibility they need.
Edge AI vs Cloud AI: Key Differences for Business
Data Processing Location
- Edge AI: Local device or on-premise system
- Cloud AI: Remote server infrastructure
Local processing means Edge AI can make decisions on the spot, which is ideal for time-sensitive applications.
Latency and Speed
- Edge AI: Near-zero lag
- Cloud AI: Dependent on network strength and server load
Edge AI is the clear winner when milliseconds matter—think autonomous vehicles or facial recognition at entry points.
Security and Privacy
- Edge AI: Keeps data close, reducing external exposure
- Cloud AI: Transmits data to the cloud, increasing surface area for threats
Edge AI supports compliance-heavy industries like healthcare and finance with better data control.
Cost and Infrastructure
- Edge AI: Upfront investment in edge hardware
- Cloud AI: Pay-as-you-go model, but may scale up over time
Cloud AI may seem cheaper at first, but Edge AI often saves money long term by reducing bandwidth, storage, and processing fees.
Connectivity Requirements
- Edge AI: Works offline
- Cloud AI: Needs constant internet access
For remote sites or field operations, Edge AI is the more reliable choice.
How to Choose Between Edge and Cloud AI for Business
Choosing the right AI infrastructure depends on your goals and constraints. Here’s a breakdown:
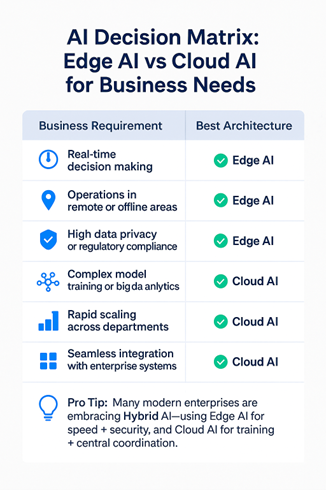
Ask Yourself:
- Do you need real-time decisions at the point of data capture?
- Are your systems located in areas with weak connectivity?
- Is data sensitivity a key concern?
- Do you plan to scale AI across multiple locations?
Need Help Choosing the Right AI Model?
Get a free 30-minute AI infrastructure consultation with our AI strategy team.
Real-World Example: Manufacturing Visual Inspection
A mid-sized auto parts manufacturer was struggling with delayed defect detection using a cloud-based vision system. They switched to Edge AI, embedding vision models directly into cameras on the assembly line.
The results?
- Defect response time dropped from 5 seconds to under 100 milliseconds
- Monthly cloud storage costs were reduced by 70%
- Production errors fell by 40% in 3 months
This is the power of picking the right AI model from the start.
Not sure if Edge or Cloud AI is right for you?
Chat with our AI experts to explore the best setup for your business.
Hybrid AI: The Best of Both Worlds?
Many enterprises are blending Edge and Cloud AI to maximize flexibility.
Hybrid AI Advantages
- Use Edge AI for immediate decision-making on devices
- Use Cloud AI for model training, long-term data storage, and analytics
- Deploy updates to edge devices via cloud pipelines
For many businesses, the smartest path forward isn’t picking a side—it’s combining both. A hybrid approach lets you deploy AI where it performs best: on the edge for speed and security, and in the cloud for model training and coordination. For instance, a logistics company might use Edge AI for on-vehicle monitoring and route optimisation in real time, while relying on Cloud AI to analyse trends across its entire fleet. This layered strategy is gaining traction because it avoids trade-offs and instead builds a more adaptable, future-ready ecosystem.
This hybrid setup helps businesses remain agile without sacrificing speed or insight.
Frequently Asked Questions About Edge AI vs Cloud AI
Q: Which is faster—Edge AI or Cloud AI?
A: Edge AI is faster for real-time tasks because it processes data locally, while Cloud AI depends on internet connectivity and server load.
Q: Is Edge AI more secure than Cloud AI?
A: Yes. Since Edge AI keeps data on local devices, it reduces the risk of exposure during transmission, making it ideal for privacy-sensitive industries.
Q: Can you combine Edge AI and Cloud AI?
A: Absolutely. A hybrid AI setup lets you process time-sensitive tasks locally while leveraging cloud resources for training and analytics.
Q: What is the best AI architecture for remote field operations?
A: Edge AI is best for remote or low-connectivity environments, offering offline functionality and on-device decision-making.
Final Take: Your AI Infrastructure Will Define Your Next Decade
When deciding between Edge AI and Cloud AI, align your infrastructure with business outcomes like speed, scalability, and data control. Whether it’s the instant decision-making of Edge AI or the powerful capabilities of Cloud AI, align your choice with your goals, not just the tech specs.
Thinking of using Edge AI, Cloud AI or a Hybrid AI solution?
Book your free AI strategy session today and future-proof your operations.